Machine Learning Latest Updates: Innovations Transforming Business
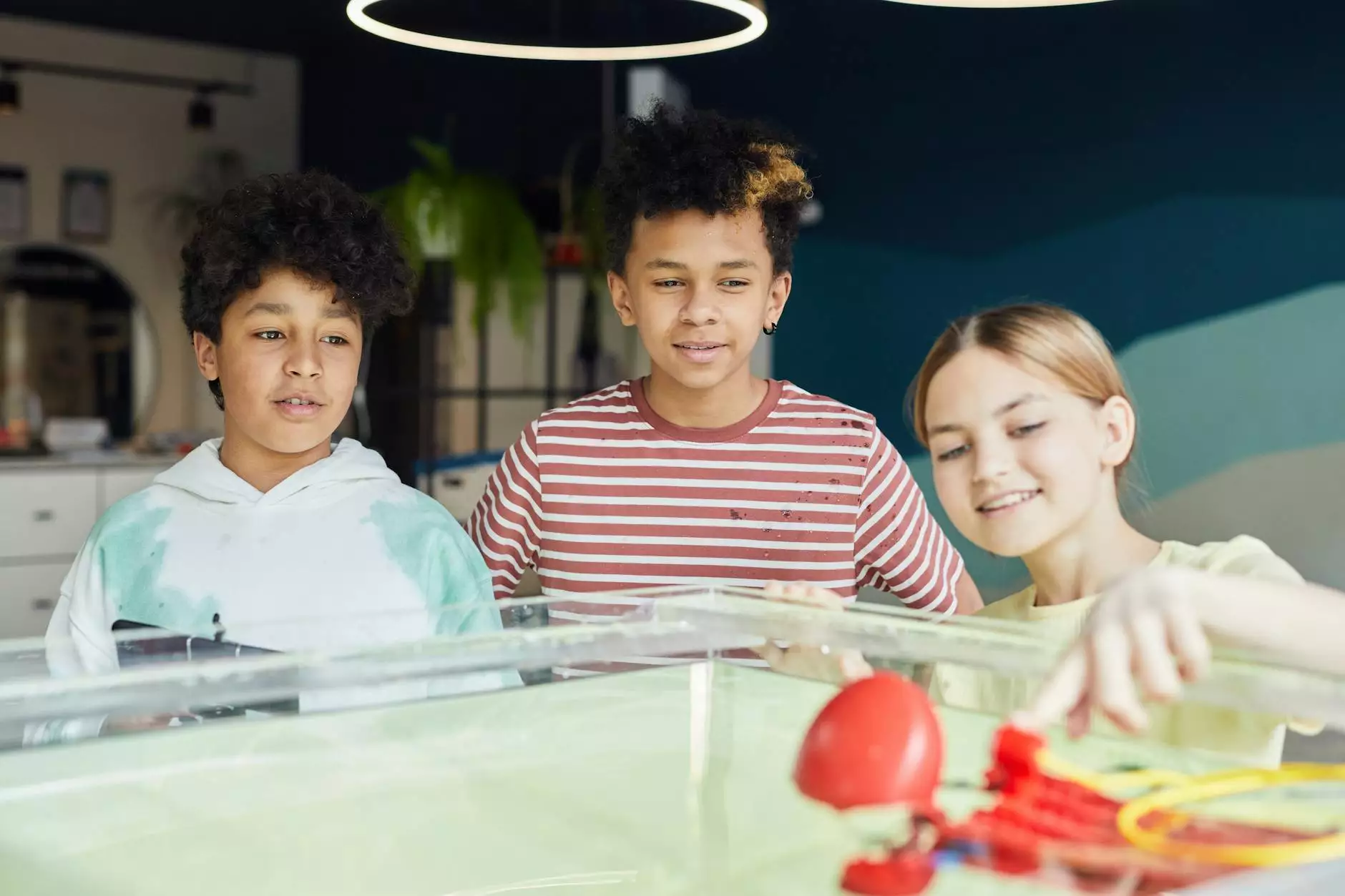
Machine Learning (ML) has evolved tremendously over the last few years, becoming an integral part of numerous industries. In today's hyper-competitive market, businesses are leveraging machine learning latest updates to stay ahead of the curve. This article explores these advancements, focusing on their application in various sectors and how they can contribute to business growth and efficiency.
The Evolution of Machine Learning
Machine Learning refers to the branch of artificial intelligence that enables computer systems to learn and improve from experience without being explicitly programmed. The journey of ML has seen significant advancements:
- Early Days: Initial algorithms took shape in the mid-20th century, focusing on basic pattern recognition.
- Statistical Methods: The late 1990s and early 2000s introduced sophisticated statistical models and analysis techniques.
- Deep Learning: The renaissance in computing power around 2010 gave rise to deep learning, revolutionizing fields like image and speech recognition.
Key Innovations in Machine Learning
The landscape of machine learning is constantly evolving. Here are some of the latest updates that are shaping the future of this technology:
1. Automated Machine Learning (AutoML)
One of the most significant trends is the advent of AutoML. This technology automates several aspects of machine learning, making it accessible to non-experts.
- Efficiency: AutoML systems can automatically select the best model and fine-tune its parameters, freeing data scientists to focus on more complex tasks.
- Accessibility: Non-technical users can now employ machine learning models without extensive programming knowledge.
2. Transfer Learning
Transfer Learning involves taking a pre-trained model and fine-tuning it for specific tasks. This method allows businesses to apply strong models with less data, dramatically speeding up training times and reducing costs.
3. Explainable AI (XAI)
As businesses integrate ML algorithms into their operations, the demand for Explainable AI has surged. Companies are concerned about transparency and accountability in AI decision-making processes.
- Trust and Adoption: Providing explanations for AI decisions increases trust among stakeholders.
- Regulatory Compliance: Many industries need to comply with regulations requiring transparency in AI processes.
Applications of Machine Learning in Business
The impact of machine learning latest updates can be felt across various sectors. Here are some of the most notable applications:
1. Healthcare
Machine learning is revolutionizing healthcare through predictive analytics, disease detection, and personalized medicine:
- Predictive Analytics: ML algorithms analyze patient data to predict outcomes, enabling proactive interventions.
- Medical Imaging: AI algorithms can interpret images from MRIs and CT scans with remarkable accuracy, assisting doctors in diagnostics.
- Personalized Medicine: Machine learning helps tailor treatments to individual patients based on their unique genetic profiles.
2. Financial Services
The finance sector utilizes machine learning for risk assessment, fraud detection, and algorithmic trading:
- Risk Management: ML models assess risk levels by analyzing historical data and market trends.
- Fraud Detection: Banks and financial institutions employ machine learning to detect suspicious activities and reduce losses.
- Algorithmic Trading: Automated trading systems use ML to analyze stock market trends and execute trades at optimal times.
3. Retail
In retail, businesses use machine learning to enhance customer experiences and optimize supply chains:
- Personalized Recommendations: E-commerce platforms leverage ML to analyze customer behavior, offering personalized product suggestions.
- Inventory Management: ML algorithms forecast demand accurately, helping retailers stock efficiently and minimize costs.
4. Manufacturing
The manufacturing industry employs machine learning for predictive maintenance, quality control, and supply chain optimization:
- Predictive Maintenance: ML analyzes equipment data to predict failures before they occur, reducing downtime.
- Quality Control: Automated systems powered by ML continuously monitor production processes to maintain quality standards.
Challenges and Considerations
Despite its numerous benefits, incorporating machine learning poses challenges for businesses:
1. Data Privacy and Security
As businesses collect vast amounts of data, concerns regarding privacy and security are paramount. Companies must ensure compliance with regulations such as GDPR and HIPAA.
2. Lack of Talent
There is a notable shortage of qualified machine learning professionals. Businesses must invest in training and development to bridge the talent gap.
3. Integration with Existing Systems
Integrating new ML systems with existing business processes can be complex. It's crucial for organizations to plan their implementation strategies meticulously.
Future Trends in Machine Learning
The field of machine learning is rapidly advancing. Here are some trends to watch:
1. Federated Learning
Federated Learning is a new paradigm where machine learning models learn across multiple decentralized devices without sharing data. This enhances privacy and security, making it attractive for businesses concerned about sensitive information.
2. Enhanced Natural Language Processing (NLP)
With continued improvements in NLP, businesses can expect more sophisticated chatbots and virtual assistants that enhance customer interactions.
3. Machine Learning Operations (MLOps)
As machine learning becomes more widespread, the need for MLOps arises—tools and practices that allow for the smooth deployment and monitoring of ML models. This streamlines workflows and ensures models remain performant and relevant over time.
Conclusion
As we delve into the machine learning latest updates, it is clear that this technology is not just a trend but a cornerstone of modern business strategies. Organizations that embrace these innovations will not only improve their operational efficiencies but also position themselves to thrive in an increasingly data-driven world. By understanding and leveraging the full potential of machine learning, businesses can unlock new pathways for growth, customer engagement, and efficiency, ensuring they remain at the forefront of their industries.
For more insights into how machine learning can transform your business, visit machinelearningconsulting.net.